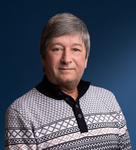
COUTURE Patrice
- Centre Eau Terre Environnement, Institut national de la recherche scientifique, Québec, Canada
- Aquatic ecotoxicology, Bioaccumulation/biomagnification, Bioassays, Biomarkers, Biomonitoring, Environmental pollution, Legacy and emerging contaminants, Marine ecotoxicology
- recommender, manager
Recommendations: 2
Reviews: 2
Recommendations: 2
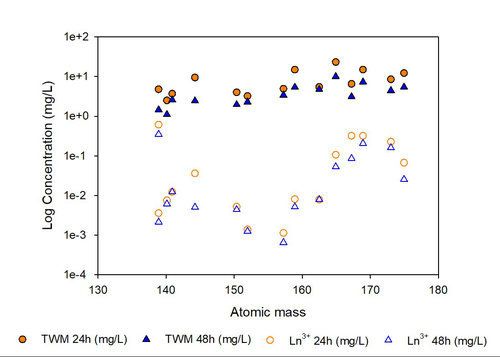
Ecotoxicity of lanthanides to Daphnia magna: insights from elemental behavior and speciation in a standardized test medium
Lanthanide atomic mass and chemical behaviour in solution influence their solubility and ecotoxicity for Daphnia magna: Implications for risk assessment of aquatic organisms
Recommended by Patrice Couture based on reviews by Carrie J. Rickwood and 1 anonymous reviewerThe demand for lanthanides (LN) has seen a steady increase and is anticipated to continue to grow. Due to their unique properties, they have become essential in key components of new technologies, such as batteries, wind turbines, electronic components and other devices needed to facilitate energy transition away from fossil fuels. These elements are also increasingly used in a range of new technologies, including medical applications and telecommunication. In this context, the concentrations of lanthanides are expected to increase in freshwater environments (Gwenzi et al., 2018). Our limited knowledge about the risk that they pose to organisms limits our ability to develop guidelines for environmental protection. Research on this issue has so far been hindered by the peculiar properties of lanthanides, that tend to form insoluble precipitates when added in standard ecotoxicological test media (Blinova et al., 2018). This and other challenges of studying lanthanide toxicity were addressed in this in-depth study that leaves few stones unturned.
The study by Vignati and colleagues (2024) is the first to investigate the acute toxicity of all LN, with the exception of promethium, a radioactive element, on Daphnia magna, a model test species, following the ISO 6341 (2012) norm. The authors designed their study to generate data useable for the development of risk assessment guidelines for the LN series and to generate data-based recommendations for future studies on LN ecotoxicity. They exposed daphnids to nine to ten dilutions of all tested LN in a medium and carried out 48-hour acute immobilization assays. Initial and final pH was measured along with concentrations of LN in the test solutions sampled at various intervals by ICP-MS. This data allowed calculation of LN speciation, performed using VisualMinteq software. Effect concentrations were also calculated using different metrics based on initial (nominal), time-averaged or modelled LN3+ exposure concentrations.
In their multi-faceted investigation, the authors reported several observations that clearly contribute to a better understanding of the ecotoxicity of LN to aquatic organisms and provide useful advice for future studies, briefly summarized here. Proper characterization of exposure concentrations is a key in any ecotoxicological study. Their project shows that even for a short, 48 h exposure, LN concentrations decrease due to a combination of precipitation and, possibly, adsorption. The concentration decrease was inversely proportional to the LN atomic mass, which may reduce the analytical requirements for future studies using the same test medium. The addition of LN to the test medium also modified pH and a detailed hypothesis is formulated to explain this phenomenon that has implications for ecotoxicological endpoints. Conclusions on LN ecotoxicity drawn in this study are based on experimental data and on extensive thermodynamic speciation modeling. The values of EC50 presented in the study varied by several order of magnitude depending on the chosen exposure metric, underscoring the urgent need for consensus-building on this issue across the research community. The authors also provide a comparison of their conclusions on EC50 values for daphnids with the limited data available in the literature, further validating their data with cautions carefully laid out about experimental design. The paper concludes with a list of seven caveats that should be considered both for regulators who will want to use the data presented in the paper for environmental LN concentrations regulations and for future studies. These caveats highlight the importance of considering LN speciation and chemical behavior during ecotoxicity assays, their influence on exposure concentrations, and their importance for risk assessment. They also reiterate that since LN concentrations in filtered water collected in the field are not directly comparable to EC50 values derived from laboratory studies using total or free LN3+ concentrations, an effort must be made to harmonize the methods of LN concentration measurements in field and laboratory studies. Overall, this paper may be one of the most rigorous studies in the current literature about LN ecotoxicity in freshwater systems. In its approach, it sets a precedent for future studies aiming at generating EC50 values or other toxicological endpoints of inorganic contaminants. The paper, carefully reviewed by Carrie Rickwood and by an anonymous reviewer, is a major contribution towards our understanding of LN ecotoxicity.
References
Blinova, I., Lukjanova, A., Muna, M., Vija, H., & Kahru, A. (2018). Evaluation of the potential hazard of lanthanides to freshwater microcrustaceans. Sci. Tot. Environ. 642 :1100-1107. https://doi.org/10.1016/j.scitotenv.2018.06.155
Gwenzi, W., Mangori, L., Danha, C., Chaukura, N, Dunjana, N., Sanganyado, E. (2018). Sources, behaviour, and environmental and human health risks of high technology rare earth elements as emerging contaminants. Sci. Total Environ., 636:299-313. https://doi.org/10.1016/j.scitotenv.2018.04.235
ISO. (2012). Water quality — Determination of the inhibition of the mobility of Daphnia magna Straus (Cladocera, Crustacea) — Acute toxicity test (norm 6341). https://www.iso.org/standard/54614.html
Vignati, D.A.L., Martin, L.A., Poirier, L., Zalouk-Vergnoux, A., Fouque, C., Clément, B., Hissler, C., Cossu-Leguille, C. (2024). Ecotoxicity of lanthanides to Daphnia magna: insights from elemental behavior and speciation in a standardized test medium. Ver.3 peer-reviewed and recommended by Peer Community In Ecotoxicology and Environmental Chemistry. https://hal.science/LIEC-UL/hal-04302491v3
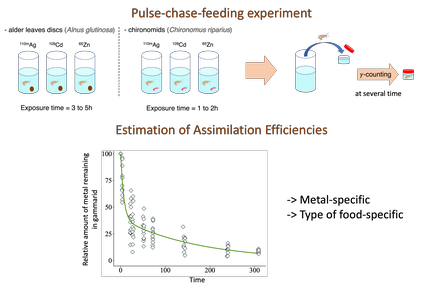
Assimilation efficiencies and elimination rates of silver, cadmium and zinc accumulated by trophic pathway in Gammarus fossarum
Food type influences dietary metal uptake and elimination in Gammarus fossarum
Recommended by Patrice Couture based on reviews by Davide Anselmo Luigi Vignati and Valentin GeslinGiven their narrow associations with human civilization, including urban, agricultural and industrial settings, freshwater systems worldwide are primary recipients of contaminants from anthropogenic origins, threatening biodiversity (Dudgeon 2019). Freshwater invertebrates are typically abundant in these environments. They are easily sampled, and several species can also be raised in the laboratory. Furthermore, they have the propensity to accumulate contaminants from their environments through both aqueous and dietary routes. These traits make them ideally suited as bioindicators of environmental contamination and for the study of the mechanisms of contaminant uptake and effects. Therefore, over the last decades, several studies have investigated the bioaccumulation and toxicity of a wide range of organic and inorganic contaminants. Knowledge of the relative importance of the aqueous and dietary exposure routes is key to understanding the processes involved in contaminant uptake and organismal and ecological consequences. Although the mechanisms of aqueous uptake have received much attention in recent literature, those associated with dietary uptake are far less known. This is the case for species commonly used for biomonitoring environmental contamination such as the amphipod Gammarus fossarum, and for metals of major concern for the Water Framework Directive (WFD) such as Ag, Cd and Zn.
To address these knowledge gaps, Gestin et al (2024) investigated the assimilation efficiency (AE) of Ag, Cd and Zn from two contrasting types of food, one plant (alder leaves) and one invertebrate (Chironomus riparius larvae) for gammarids using a pulse-chase-feeding method in a laboratory setting. Food was radiolabeled and fed for a short period to gammarids (3 to 5 hours for alder leaves and 1 hour for chironomid larvae), after which they were left to depurate for 14 days, during which period they were fed with uncontaminated alder leaves. During the depuration period, gammarids were monitored to follow radioactivity using a gamma counter. A nonlinear least squares modelling approach was used to estimate assimilation efficiencies and elimination rates of the metals from each food source.
From this data, the authors concluded that Cd was assimilated with a higher efficiency, followed by Zn, with Ag showing the lowest AE. Their data also showed that the AE of Cd and Zn was higher when gammarids were fed alder leaves compared to chironomid larvae. In contrast, elimination rates were not different among metals but varied between food types, with metals from chironomids being eliminated more slowly than those from alder leaves. Elimination rate and AE of Ag could not be determined for gammarids fed chironomid larvae, due to undetectable radioactivity. This study highlights that the assimilation and elimination rates of metals ingested from food depend on their chemical properties and on the way the metals are stored in prey. The data needs to be interpreted by taking into consideration that since chironomid larvae were live prey, they could internalize the metals and make it more difficult to accumulate for the gammarid consumer, compared to the inert matrix of dead alder leaves. This study will contribute to improving toxicokinetic models needed to improve regulatory guidelines for metals in freshwater systems.
References
Dudgeon, D. (2019). Multiple threats imperil freshwater biodiversity in the Anthropocene. Current Biology 29(19):R960-R967. https://doi.org/10.1016/j.cub.2019.08.002
Gestin, O., Lopes, C., Delorme, N., Garnero, L., Geffard, O., Lacoue-Labarthe, T. (2024). Assimilation efficiencies and elimination rates of silver, cadmium and zinc accumulated by trophic pathway in Gammarus fossarum. bioRxiv, 2023.07.14.549054, ver.4 peer-reviewed and recommended by Peer Community In Ecotoxicology and Environmental Chemistry. https://doi.org/10.1101/2023.07.14.549054
Reviews: 2
Identifying pesticide mixtures at country-wide scale
An original approach for the identification of relevant pesticides mixtures at nationwide scale
Recommended by Pierre Labadie based on reviews by Patrice Couture and Clémentine FRITSCHOver the last decades, pesticides have been massively used in agriculture and their impacts on both the environment and human health are a major growing concern (Humann-Guilleminot et al., 2019; 2019 Boedeker et al., 2020). Improving the prediction of wildlife exposure to pesticides and the associated impacts on ecosystems is therefore crucial. In general, ecotoxicological studies addressing the effects of pesticides include compounds that are selected based on general use over large areas (e.g. regions, country) or specific crop types. Such a selection does not necessarily reflect the mixtures to which species of wildlife are exposed in a particular ecosystem.
In this context, Cairo et al. (2023) present an original approach to identify relevant mixtures of current-use pesticides. Their approach relies on public data concerning pesticide sales and cropping, available at a nationwide scale in France and at a relatively high resolution (i.e. postcode of the buyer). Based on a number of clearly exposed and discussed assumptions (e.g. “pesticides were used in the year of purchase and in the postcode of purchase”), their approach allowed for identifying 18 groups that were discriminated by a reduced number of pesticides. Some compounds were found in most or all groups and were termed “core substances” (e.g. deltamethrin and lambda-cyhalothrin). Other compounds, however, were associated with a limited number of groups and termed “discriminant substances” (e.g. boscalid and epoxiconazole).
The authors identified groups of molecules that are probably associated with the same mixtures, which warrants the investigation of potential synergetic effects. In addition, their approach allowed for the identification of areas where aquatic biota may be exposed to similar mixtures, which is might prove of interest to further investigate in situ the actual impacts of pesticide mixtures on ecosystems. Note that the approach taken by the authors might be applied by others in other countries, provided a database of pesticide sales is available.
REFERENCES
Boedeker W, Watts M, Clausing P, Marquez E (2020) The global distribution of acute unintentional pesticide poisoning: estimations based on a systematic review. BMC Public Health, 20, 1875. https://doi.org/10.1186/s12889-020-09939-0
Cairo M, Monnet A-C, Robin S, Porcher E, Fontaine C (2023) Identifying pesticide mixtures at country-wide scale. HAL, ver. 2 peer-reviewed and recommended by Peer Community in Ecotoxicology and Environmental Chemistry. https://hal.science/hal-03815557
Humann-Guilleminot S, Tassin de Montaigu C, Sire J, Grünig S, Gning O, Glauser G, Vallat A, Helfenstein F (2019) A sublethal dose of the neonicotinoid insecticide acetamiprid reduces sperm density in a songbird. Environmental Research, 177, 108589. https://doi.org/10.1016/j.envres.2019.108589
Machine learning models based on molecular descriptors to predict human and environmental toxicological factors in continental freshwater
Predicting characterization factors of chemical substances from a set of molecular descriptors based on machine learning algorithms
Recommended by Sandrine CHARLES based on reviews by Patrice Couture, Sylvain Bart, Dominique Lamonica and 2 anonymous reviewersToday, thousands of chemical substances are released into the environment because of human activities. It is thus crucial to identify all relevant chemicals that contribute to toxic effects on living organisms, also potentially disturbing the community functioning and the ecosystem services that flow from them. Once identified, chemical substances need to be associated with ecotoxicity factors. Nevertheless, getting such factors usually requires time-, resources- and animal-costly experiments that it should be possible to avoid. In this perspective, modelling approaches may be particularly helpful if they rely on easy-to-obtain information to be used as predictive variables.
Within this context, the paper of Servien et al. (2022) illustrates the use of machine learning algorithms to predict toxicity and ecotoxicity factors that were missing for a collection of compounds. Their modelling approach involve a collection of molecular descriptors as input variables. A total of 40 molecular descriptors were extracted from the TyPol database (Servien et al., 2014) as those describing the best how organic compounds behave within the environment. These molecular descriptors also have the advantage to be easily quantifiable for new chemical substances under evaluation. The performances of the proposed models were systematically checked and compared to the classical linear partial least square method, based on the calculation of the absolute error (namely, the difference between prediction and true value). This finally led to different best models (that is associated to the lowest median absolute error) according to the classification of the 526 compounds comprised in the TyPol database in five clusters. These five clusters of different sizes gather chemical substances with different but specific molecular characteristics, also corresponding to different estimates of the characterization factors both in their median and within-variability.
In a final step, predictions of characterization factors were performed for 102 missing values in the USEtox® database (Rosenbaum et al., 2008) but also referenced in TyPol. This paper highlights that the molecular descriptors that explain the most the toxicity of the chemical substances in each cluster strongly differ. Nevertheless, these predictions, whatever the cluster, appear precise enough to be considered as relevant despite everything.
As a conclusion, this paper is a promising proof-of-concept in using machine learning modelling to go beyond some constraints around the toxicity evaluation of chemical substances, especially handling non-linearities and data-demanding calculations, in in an ever-changing world that is gradually depleting its resources without sufficient concern for the short-term risks to the environment and human health.
References
Rosenbaum RK, Bachmann TM, Gold LS, Huijbregts MAJ, Jolliet O, Juraske R, Koehler A, Larsen HF, MacLeod M, Margni M, McKone TE, Payet J, Schuhmacher M, van de Meent D, Hauschild MZ (2008) USEtox—the UNEP-SETAC toxicity model: recommended characterisation factors for human toxicity and freshwater ecotoxicity in life cycle impact assessment. The International Journal of Life Cycle Assessment, 13, 532. https://doi.org/10.1007/s11367-008-0038-4
Servien R, Latrille E, Patureau D, Hélias A (2022) Machine learning models based on molecular descriptors to predict human and environmental toxicological factors in continental freshwater. bioRxiv, 2021.07.20.453034, ver. 6 peer-reviewed and recommended by Peer Community in Ecotoxicology and Environmental Chemistry. https://doi.org/10.1101/2021.07.20.453034
Servien R, Mamy L, Li Z, Rossard V, Latrille E, Bessac F, Patureau D, Benoit P (2014) TyPol – A new methodology for organic compounds clustering based on their molecular characteristics and environmental behavior. Chemosphere, 111, 613–622. https://doi.org/10.1016/j.chemosphere.2014.05.020