Latest recommendations
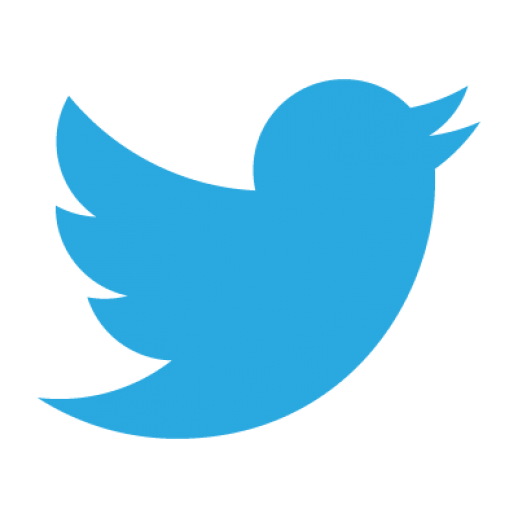
Id | Title * | Authors * | Abstract * | Picture * | Thematic fields * | Recommender | Reviewers | Submission date | |
---|---|---|---|---|---|---|---|---|---|
30 Nov 2022
![]() Chemical effects on ecological interactions within a model-experiment loopDominique LAMONICA, Sandrine CHARLES, Bernard CLÉMENT, Christelle LOPES https://doi.org/10.1101/2022.05.24.493191A model-experiment loop to optimise data requirements for ecotoxicological risk assessment with mesocosmsRecommended by Volker Grimm based on reviews by Charles Hazlerigg and Peter VermeirenIn Ecotoxicology, the toxicity of chemicals is usually quantified for individuals under laboratory conditions, while in reality individuals interact with other individuals in populations and communities, and are exposed to conditions that vary in space and time. Micro- and mesocosm experiments are therefore used to increase the ecological realism of toxicological risk assessments. Such experiments are, however, labour-intensive, costly, and cannot, due to logistical reasons, implement all possible factors or interests (Henry et al. 2017). Moreover, as such experiments often include animals, the number of experiments performed has to be minimized to reduce animal testing as much as possible. Modelling has therefore been suggested to complement such experiments (Beaudoin et al. 2012). Still, the population models of the species involved need to be parameterized and can thus require a large amount of data. However, how much data are actually needed is usually unclear. Lamonica et al. (2022) therefore focus on the challenge of “taking the most of experimental data and reducing the amount of experiments to perform”. Their ultimate goal is to reduce the number of experiments to parameterize their model of a 3-species mesocosm, comprised of algae, duckweed, and water fleas, sufficiently well. For this, experiments with one, two or three species, with different cadmium concentrations and without cadmium, are performed and used to parameterize, using the Bayesian Monte Carlo Markov Chain (MCMC) method, the model. Then, different data sets omitting certain experiments are used for the same parameterization procedure to see which data sets, and hence experiments, might possibly be omitted when it comes to parameterizing a model that would be precise enough to predict the effects of a toxicant. The authors clearly demonstrate the added value of the approach, but also discuss limits to the transferability of their recommendations. Their manuscript presents a useful and inspiring illustration of how in the future models and experiments should be combined in an integrated, iterative process. This is in line with the current “Destination Earth” initiative of the European Commission, which aims at producing “digital twins” of different environmental sectors, where the continuous mutual updating of models and monitoring designs is the key idea. The authors make an important point when concluding that “data quality and design are more beneficial for modelling purpose than quantity. Ideally, as the use of models and big data in ecology increases […], modellers and experimenters could collaboratively and profitably elaborate model-guided experiments.” References Beaudouin R, Ginot V, Monod G (2012) Improving mesocosm data analysis through individual-based modelling of control population dynamics: a case study with mosquitofish (Gambusia holbrooki). Ecotoxicology, 21, 155–164. https://doi.org/10.1007/s10646-011-0775-1 Henry M, Becher MA, Osborne JL, Kennedy PJ, Aupinel P, Bretagnolle V, Brun F, Grimm V, Horn J, Requier F (2017) Predictive systems models can help elucidate bee declines driven by multiple combined stressors. Apidologie, 48, 328–339. https://doi.org/10.1007/s13592-016-0476-0 Lamonica D, Charles S, Clément B, Lopes C (2022) Chemical effects on ecological interactions within a model-experiment loop. bioRxiv, 2022.05.24.493191, ver. 6 peer-reviewed and recommended by Peer Community in Ecotoxicology and Environmental Chemistry. https://doi.org/10.1101/2022.05.24.493191 | Chemical effects on ecological interactions within a model-experiment loop | Dominique LAMONICA, Sandrine CHARLES, Bernard CLÉMENT, Christelle LOPES | <p style="text-align: justify;">We propose in this paper a method to assess the effects of a contaminant on a micro-ecosystem, integrating the population dynamics and the interactions between species. For that, we developed a dynamic model to desc... | ![]() | Aquatic ecotoxicology, Environmental risk assessment, Modelling, Species interactions-webs | Volker Grimm | Charles Hazlerigg, Peter Vermeiren | 2022-05-30 11:05:59 | View |
18 Jan 2022
Machine learning models based on molecular descriptors to predict human and environmental toxicological factors in continental freshwaterRémi Servien, Eric Latrille, Dominique Patureau, Arnaud Hélias https://doi.org/10.1101/2021.07.20.453034Predicting characterization factors of chemical substances from a set of molecular descriptors based on machine learning algorithmsRecommended by Sandrine CHARLESToday, thousands of chemical substances are released into the environment because of human activities. It is thus crucial to identify all relevant chemicals that contribute to toxic effects on living organisms, also potentially disturbing the community functioning and the ecosystem services that flow from them. Once identified, chemical substances need to be associated with ecotoxicity factors. Nevertheless, getting such factors usually requires time-, resources- and animal-costly experiments that it should be possible to avoid. In this perspective, modelling approaches may be particularly helpful if they rely on easy-to-obtain information to be used as predictive variables. Within this context, the paper of Servien et al. (2022) illustrates the use of machine learning algorithms to predict toxicity and ecotoxicity factors that were missing for a collection of compounds. Their modelling approach involve a collection of molecular descriptors as input variables. A total of 40 molecular descriptors were extracted from the TyPol database (Servien et al., 2014) as those describing the best how organic compounds behave within the environment. These molecular descriptors also have the advantage to be easily quantifiable for new chemical substances under evaluation. The performances of the proposed models were systematically checked and compared to the classical linear partial least square method, based on the calculation of the absolute error (namely, the difference between prediction and true value). This finally led to different best models (that is associated to the lowest median absolute error) according to the classification of the 526 compounds comprised in the TyPol database in five clusters. These five clusters of different sizes gather chemical substances with different but specific molecular characteristics, also corresponding to different estimates of the characterization factors both in their median and within-variability. In a final step, predictions of characterization factors were performed for 102 missing values in the USEtox® database (Rosenbaum et al., 2008) but also referenced in TyPol. This paper highlights that the molecular descriptors that explain the most the toxicity of the chemical substances in each cluster strongly differ. Nevertheless, these predictions, whatever the cluster, appear precise enough to be considered as relevant despite everything. As a conclusion, this paper is a promising proof-of-concept in using machine learning modelling to go beyond some constraints around the toxicity evaluation of chemical substances, especially handling non-linearities and data-demanding calculations, in in an ever-changing world that is gradually depleting its resources without sufficient concern for the short-term risks to the environment and human health. References Rosenbaum RK, Bachmann TM, Gold LS, Huijbregts MAJ, Jolliet O, Juraske R, Koehler A, Larsen HF, MacLeod M, Margni M, McKone TE, Payet J, Schuhmacher M, van de Meent D, Hauschild MZ (2008) USEtox—the UNEP-SETAC toxicity model: recommended characterisation factors for human toxicity and freshwater ecotoxicity in life cycle impact assessment. The International Journal of Life Cycle Assessment, 13, 532. https://doi.org/10.1007/s11367-008-0038-4 Servien R, Latrille E, Patureau D, Hélias A (2022) Machine learning models based on molecular descriptors to predict human and environmental toxicological factors in continental freshwater. bioRxiv, 2021.07.20.453034, ver. 6 peer-reviewed and recommended by Peer Community in Ecotoxicology and Environmental Chemistry. https://doi.org/10.1101/2021.07.20.453034 Servien R, Mamy L, Li Z, Rossard V, Latrille E, Bessac F, Patureau D, Benoit P (2014) TyPol – A new methodology for organic compounds clustering based on their molecular characteristics and environmental behavior. Chemosphere, 111, 613–622. https://doi.org/10.1016/j.chemosphere.2014.05.020 | Machine learning models based on molecular descriptors to predict human and environmental toxicological factors in continental freshwater | Rémi Servien, Eric Latrille, Dominique Patureau, Arnaud Hélias | <p style="text-align: justify;">It is a real challenge for life cycle assessment practitioners to identify all relevant substances contributing to the ecotoxicity. Once this identification has been made, the lack of corresponding ecotoxicity facto... | Aquatic ecotoxicology, Ecosystem Health, Environmental pollution, Modelling | Sandrine CHARLES | 2021-07-21 09:09:50 | View |