
A model-experiment loop to optimise data requirements for ecotoxicological risk assessment with mesocosms
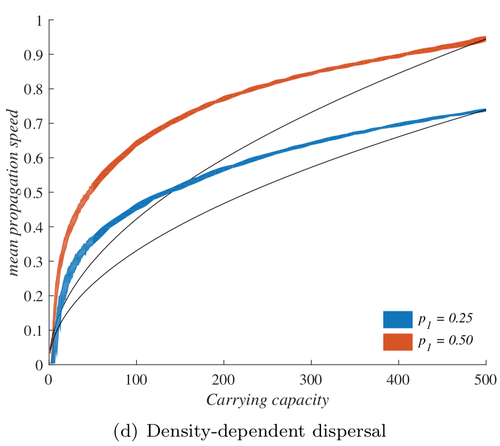
Chemical effects on ecological interactions within a model-experiment loop
Abstract
Recommendation: posted 20 October 2022, validated 30 November 2022
Grimm, V. (2022) A model-experiment loop to optimise data requirements for ecotoxicological risk assessment with mesocosms. Peer Community in Ecotoxicology and Environmental Chemistry, 100002. https://doi.org/10.24072/pci.ecotoxenvchem.100002
Recommendation
In Ecotoxicology, the toxicity of chemicals is usually quantified for individuals under laboratory conditions, while in reality individuals interact with other individuals in populations and communities, and are exposed to conditions that vary in space and time. Micro- and mesocosm experiments are therefore used to increase the ecological realism of toxicological risk assessments. Such experiments are, however, labour-intensive, costly, and cannot, due to logistical reasons, implement all possible factors or interests (Henry et al. 2017). Moreover, as such experiments often include animals, the number of experiments performed has to be minimized to reduce animal testing as much as possible.
Modelling has therefore been suggested to complement such experiments (Beaudoin et al. 2012). Still, the population models of the species involved need to be parameterized and can thus require a large amount of data. However, how much data are actually needed is usually unclear. Lamonica et al. (2022) therefore focus on the challenge of “taking the most of experimental data and reducing the amount of experiments to perform”.
Their ultimate goal is to reduce the number of experiments to parameterize their model of a 3-species mesocosm, comprised of algae, duckweed, and water fleas, sufficiently well. For this, experiments with one, two or three species, with different cadmium concentrations and without cadmium, are performed and used to parameterize, using the Bayesian Monte Carlo Markov Chain (MCMC) method, the model. Then, different data sets omitting certain experiments are used for the same parameterization procedure to see which data sets, and hence experiments, might possibly be omitted when it comes to parameterizing a model that would be precise enough to predict the effects of a toxicant.
The authors clearly demonstrate the added value of the approach, but also discuss limits to the transferability of their recommendations. Their manuscript presents a useful and inspiring illustration of how in the future models and experiments should be combined in an integrated, iterative process. This is in line with the current “Destination Earth” initiative of the European Commission, which aims at producing “digital twins” of different environmental sectors, where the continuous mutual updating of models and monitoring designs is the key idea.
The authors make an important point when concluding that “data quality and design are more beneficial for modelling purpose than quantity. Ideally, as the use of models and big data in ecology increases […], modellers and experimenters could collaboratively and profitably elaborate model-guided experiments.”
References
Beaudouin R, Ginot V, Monod G (2012) Improving mesocosm data analysis through individual-based modelling of control population dynamics: a case study with mosquitofish (Gambusia holbrooki). Ecotoxicology, 21, 155–164. https://doi.org/10.1007/s10646-011-0775-1
Henry M, Becher MA, Osborne JL, Kennedy PJ, Aupinel P, Bretagnolle V, Brun F, Grimm V, Horn J, Requier F (2017) Predictive systems models can help elucidate bee declines driven by multiple combined stressors. Apidologie, 48, 328–339. https://doi.org/10.1007/s13592-016-0476-0
Lamonica D, Charles S, Clément B, Lopes C (2022) Chemical effects on ecological interactions within a model-experiment loop. bioRxiv, 2022.05.24.493191, ver. 6 peer-reviewed and recommended by Peer Community in Ecotoxicology and Environmental Chemistry. https://doi.org/10.1101/2022.05.24.493191
The recommender in charge of the evaluation of the article and the reviewers declared that they have no conflict of interest (as defined in the code of conduct of PCI) with the authors or with the content of the article. The authors declared that they comply with the PCI rule of having no financial conflicts of interest in relation to the content of the article.
Reviewed by Charles Hazlerigg, 18 Oct 2022
The changes and clarifications that the authors have made to their manuscript have addressed my previous comments and enhanced the readability of the paper.
As such, my recommendation is to accept for publication.
https://doi.org/10.24072/pci.ecotoxenvchem.100002.rev21Reviewed by Peter Vermeiren, 20 Sep 2022
Dear authors,
Thank you for the carefully considering all my suggestions; they were all adequately addressed. The paper is much improved in clarity. I have no further comments.
https://doi.org/10.24072/pci.ecotoxenvchem.100002.rev22Evaluation round #1
DOI or URL of the preprint: https://doi.org/10.1101/2022.05.24.493191
Version of the preprint: 4
Author's Reply, 14 Sep 2022
Decision by Volker Grimm, posted 20 Oct 2022
This is an interesting study where the ultimate goal is to reduce the number of experiments to parameterize a 3-species mesocosm model sufficiently well. For this, experiments with one, two or three species, with different cadmium concentrations and without cadmium, are performed and used to parameterize, using the Bayesian MCMC method, the model. Then, different data sets omitting certain experiments are used for the same parameterization procedure to see which data sets, and hence experiments, might possibly be omitted when it comes to parameterize a model that would be precise enough to predict effects of a toxicant.
Both reviewers found the study interesting and scientifically sound. They still raised quite a few issues that should be addressed to improve the clarity of the presentation or provide better justifications for certain designs and assumptions.
I fully agree with the reviewers' assessments. I would like to add: an ODD model description should be complete by itself and thus not require that readers have to dig for relevant information in other papers. Here, quite a few references are made, unspecifically, to Lamonica et al. 2016a. It cannot be that much work to just copy and paste the relevant information in the current ODD.
Moreover, although I see that it can be interesting to see which kind of experiments are needed to parameterize a "full system" sufficiently well, do these insights not strongly depend on the species, experimental setup, and toxicant used? I do not fully understand how specific insights into which experiments might left out can really help us? Once you have the model fully parameterized with the full data set, there is actually no need to go back and use reduced data sets. So, for what kind of questions, or systems, can the insights gained on the relevance of specific experiments help?
Reviewed by Charles Hazlerigg, 28 Jun 2022
Reviewed by Peter Vermeiren, 29 Jun 2022
I enjoyed reading this paper because it attempts to make a concrete link between experimental study design and the use of data in modelling. Additionally, the model itself, considering the population dynamics and interactions between 3 species under cadmium exposure is interesting.
There are some general issues which remain unclear to me after reading the manuscript
- Why chose the 4 reduced datasets, what is the rationale for them. I can imagine that it is easier to maintain single species lab experiments than a microcosm. Hence, omitting experiments that require additional microcosms might be a practical (perhaps also financial) benefit. Likewise, leaving out some of the lower exposure concentrations could be a way to reduce animal testing without losing the signal of cadmium effects (which might be assumed to manifest itself at higher concentrations?). Adding these kinds of “rationales” of why the omitted data were chose would be helpful.
- It is not clear how the final recommendation came to be formulated L 440 – 451, about which datasets are best needed to inform modelling work. In fact, how transferable is this approach to inform study design to other compounds or species sets? For example, if an endocrine disrupting contaminant was tested which perhaps has a strong non-linear effect, including effects at low concentrations, would you still get to the same conclusions about omitting low concentration data? Discussing a bit more the context in which the results and recommendations are to be placed would be very helpful.
The study seems well conducted and scientifically sound. Below I provide a number of comments and suggestions that are mainly aimed at improving the clarity of the paper. None of them, however, are major flaws.
Introduction
L. 48 – 52: This section is a bit vague, not clearly linked to the previous text, and misses some details. I suggest to extend this into a separate paragraph showing concrete examples (or references) of where models have been able to link (extrapolate) between levels of biological organisation, and specifically (related to the text above) how models have been able to explicitly account for species interactions.
L. 61 – 62: Why do more complex experimental designs resist formal optimisation?
L. 67 – 70: This sounds strange: you first need to collect data, then model these data, and then you can improve how to collect the data in the first place (after you have already collected them). Perhaps this just needs a few words at the end of L.70 ... improve the experimental design for studies with microcosm experiments with similar species and compounds (or do you think it could also be useful to give guidance on experiments with more species or under compound mixtures?)
L. 73: I find it a bit difficult to understand “direct” and “indirect” effects. One could argue that direct effects are the interactions of a pollutant with a specific target molecule. Please explain in a bit more detail, e.g. “direct effects of the contaminant on a species in isolation”, and “indirect effects via contaminant effects on species interactions”. After reading the discussion I understand it a bit better, it seems direct relates to effects on specific, modelled processes, and indirect relates to effects on state variables which then cascade to affect processes where these state variables are inputs. A clear definition at the start of the paper would be useful.
L. 71 – 76: it seems to me that there is a 3rd aim: to develop critical effect concentrations for key population regulating processes (i.e. EC50 in stress functions). In fact, on L 85-86 this is mentioned as an explicit step in the project (and a discussion is given L 362 – 389).
L. 73: “how to get back from modelling to experimentation” sounds a bit bulky. How about: “how model outcomes can inform experimental design”
L. 83: It is not clear to me how “this” permits to identify direct and indirect effects. Do you mean that you used all data, including data where species occur in isolation and where they occur as a community of 3 species, to estimate model parameters, which then allows you to identify direct and indirect effects, respectively. (or did I understand wrongly, see also previous comment L 73).
L 86. I understand that you cannot say everything at once, but it would be useful to specify which processes in order to make the text less vague and easier to follow “different processes (growth of the 3 species, survival of Dapnia, and strength of interspecies interaction)”
Experiments and observed data
L 109: does measuring the size of the daphnids affect their survival?
L 112-147: The description is nice. However, I found it helpful to put this into a table to get a clearer overview. I easily get lost reading this. I would recommend adding such a table (if not in the main manuscript, then in the appendix). In fact, while making the overview table, I noticed some missing info regarding the duration and replication of some experiments (see separate review file)
Dynamic modelling
L. 162 and 167: Please double check that these are the correct references, I have the feeling that Lamonica et al 2016 a and b have been interchanged.
L. 162. When I check Lamonica et al 2016b (or a?) it says exactly the same: “the interaction is modelled with a Lotka-Volterra type 1 model”. So, referring to the Lamonica et al 2016 paper does not assist me with further details. Perhaps you can just add a few words for clarity (e.g. something like this? “... Lotka-Volterra type I model both algal and duckweed growth rate are directly proportional to ?food?”)
L. 166 Since the interaction is an important part of this paper (i.e. the aim to disentangle direct vs. indirect effects), I would find it useful to have a bit more info (and equations) about the grazing and ingestion, rather than just a reference to another paper. (I checked the Lamonica et al 2016b (not a) paper and found a good description there, but it gets a bit much to check the supplements and two other papers to find the info needed to understand a relatively important part of the study).
L. 178: Does this sentence contradict the sentence above (L. 175: “...algae and duckweeds are competing...”), as well as the use of the Lotka-Volterra type I model for competitive interaction?
L 181 – 193: It would be good to make explicit reference to the supplements (section 1.7.6 / eqn 6). Alternatively (but this might be a personal preference), it seems a shame that the actual equations are buried in the supplements, considering that this paper is developing a model.
L. 194: “We use stochasticity...” This is quite vague, and could be done in different ways. Does this only apply to the binomial distribution for the number of daphnids? I can see in Fig S1 that there are some variances added but it is a bit hard to decipher exactly where. (This sentence about stochasticity is perhaps also a bit out of place here, as you continue to describe the deterministic model equations related to Cadmium stress in the following paragraphs. Perhaps the stochasticity deserves a section on its own in the paper (e.g. just before statistical inference)?)
L. 197-200: Are there any references to support these assumptions?
Statistical inference
L. 255: Was only the Gelman Rubin diagnostic used, or did you also do a visual check. Was there a certain criterium or cut-off used to decide if the Gelman Rubin diagnostic was sufficient?
Look-back on the experimental design
Table 2: I did not immediately understand what the Cadmium concentrations C0 – C12 were referring to, but after making the table of the datasets (see comment L 112-147), I now see that there are indeed 4 groups of experimental concentrations. So, perhaps adding the table of datasets to the paper would help others as well.
Results
L 302. Something is wrong with this sentence, the English does not make sense.
L. 331: Is this a typo, I counted 5 stress functions (5 plots per dataset)
Figure 4: Why not also display the median prediction (or mode as you mention in L. 265)?
Discussion
L. 386: Wouldn't this be more due to the low value of bk rather than the narrowness of the posterior distribution?
L 393: It would be nice to have some quantification of how big the negative effect was, and what is considered “slight”.
L. 430: similar stress functions were obtained with dataset B and the reference dataset, but looking at the appendix, some of the parameters do differ when using dataset B. Perhaps this needs a mention and some discussion on whether or not changes in individual parameters are relevant to make recommendations regarding the design of lab experiments.
Conclusion
L. 464 – 475: I do not see a clear link between this paragraph and the paper.
Figures
Reference to the figure numbers seem off in a number of places throughout the text.
The labels in figure 4 are quite small
Download the review https://doi.org/10.24072/pci.ecotoxenvchem.100036.rev12